ADC in Silico Design Engine (AIDE)
Transforming Targets to Treatments: The quantitative path to optimal ADC design & development
Introduction
Development of antibody-drug conjugates (ADCs) begs a number of design questions - choice of target, antibody binding affinity, linker chemistry, and therapeutic payload all affect clinical benefit. The relationship between molecular design, lab-based assays, and human outcomes is nonlinear and difficult to characterize. AIDE, MetrumRG’s advanced computational ADC Quantitative Systems Pharmacology (QSP) model platform, supports informative and efficient decisions made on the path from discovery to patient access, including:
Asset Design
Simulate to optimize early clinical trials
Target & Indication Selection
Prioritize with precision, not guesswork
Clinical Design Optimization
Simulate to optimize early clinical trials
AIDE: QSP Platform Model
The AIDE platform integrates pharmacokinetic (PK) and cell-based pharmacodynamic (PD) models. The PK model component is based on a mAb physiologically-based PK (PBPK) model including target mediated drug disposition (TMDD) . The PD model is a mechanistic representation of the molecular level processes driving payload-induced cytotoxicity developed by MetrumRG. AIDE generates virtual population-based human PK and tumor response projections given inputs describing tumor biology (e.g., tissue specific target expression distributions) and ADC-specific properties (Figure 1).
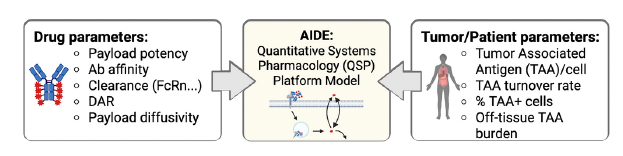
AIDE: Case Study
AIDE was trained with pre-clinical and clinical data for the HER2 ADC, T-DXd (Enhertu) to determine how it can be used to address common ADC development challenges. Projected T-DXd plasma concentrations were compared to published clinical data for breast, gastric, or gastro-oesophageal HER2+ patients treated with TDXd, demonstrating AIDE’s ability to recapitulate observed dynamics due to TMDD (Figure 2). Virtual population-based tumor dynamic responses representative of expected variability and parameter uncertainty enabled visual comparisons of projected efficacy, with results validating tumor growth inhibition (TGI) for the approved regimen of 5.4 mg/kg Q3W in HER2+ metastatic breast cancer (Figure 3).
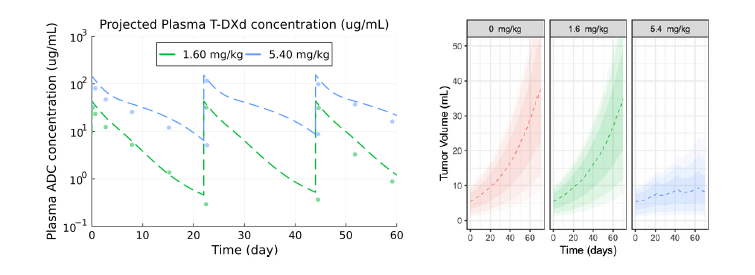
The effect of alternate tumor-associated antigen (TAA) expression levels (patient variables) and payloadpotency (ADC design parameters) on projected TGI illustrated how AIDE simulations could inform lead andtarget selection based on desired clinical outcomes (Figure 4).
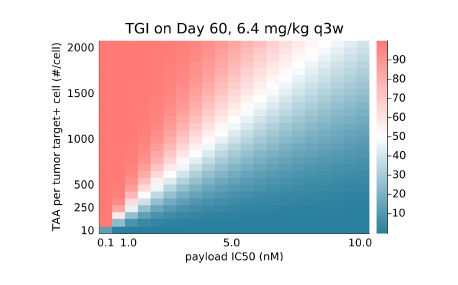
Summary
Use AIDE to inform key decision unique to your ADC program. Replace costly guesswork with clarity by quantifying the complex interplay between ADC design and patient response.